MSc thesis project proposal
[2023] Mixed-signal neuromorphic hardware for sub-10µW on-chip online learning at the edge
Neuromorphic hardware has recently been shown to enable end-to-end on-chip online learning over second-long timescales with the fully-digital ReckOn chip [1]. When the supply voltage is reduced to 0.5V, ReckOn only needs a power budget of 50µW to carry out on-chip training at the edge. While this is a record for on-chip training on temporal data, tiny autonomous sensor nodes powered by energy harvesting imply a maximum power budget of a few microwatts.
In this MSc project, we will investigate how mixed-signal design can help us further reduce the power budget of ReckOn. Indeed, while clock-based digital simulation requires state updates (and thus energy-costly memory accesses) at each integration timestep, sub-threshold analog design allows for a direct emulation of brain dynamics in real time [2]. However, the price to pay with analog design is an increase of area, as well as a higher susceptibility to noise, mismatch, and power-voltage-temperature (PVT) variations, and thus a reduction of the accuracy of the system. The goal of this MSc project is two-fold:
(i) based on a system-level power-accuracy-area evaluation, identify which computational primitives of ReckOn should be moved to the analog domain,
(ii) implement the resulting mixed-signal system, and demonstrate a new record for the power budget (ideally below 10µW).
[1] C. Frenkel, Charlotte and G. Indiveri, "ReckOn: A 28nm Sub-mm² Task-Agnostic Spiking Recurrent Neural Network Processor Enabling On-Chip Learning over Second-Long Timescales," IEEE International Solid-State Circuits Conference (ISSCC), 2022.
[2] G. Indiveri and S.-C. Liu, "Memory and information processing in neuromorphic systems." Proceedings of the IEEE, vol. 103, no. 8, pp. 1379-1397, 2015.
Requirements
For this multi-disciplinary project, background in machine learning and digital design is required. A strong background in analog design is also necessary.
Previous experience with neuromorphic architectures is not expected.
Interested students should send a motivation letter together with their CV (incl. course transcripts and grades) to Dr. Charlotte Frenkel at c.frenkel@tudelft.nl
More MSc proposals for Dr. Charlotte Frenkel will appear in the coming weeks, interested students are encouraged to reach out by e-mail to enquire about upcoming projects.
Contact
dr. Charlotte Frenkel
Electronic Instrumentation Group
Department of Microelectronics
Last modified: 2023-12-02
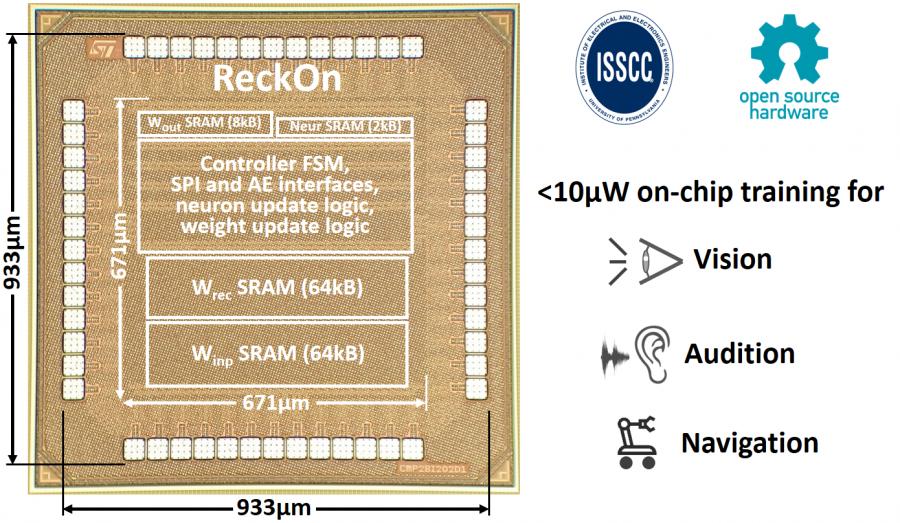